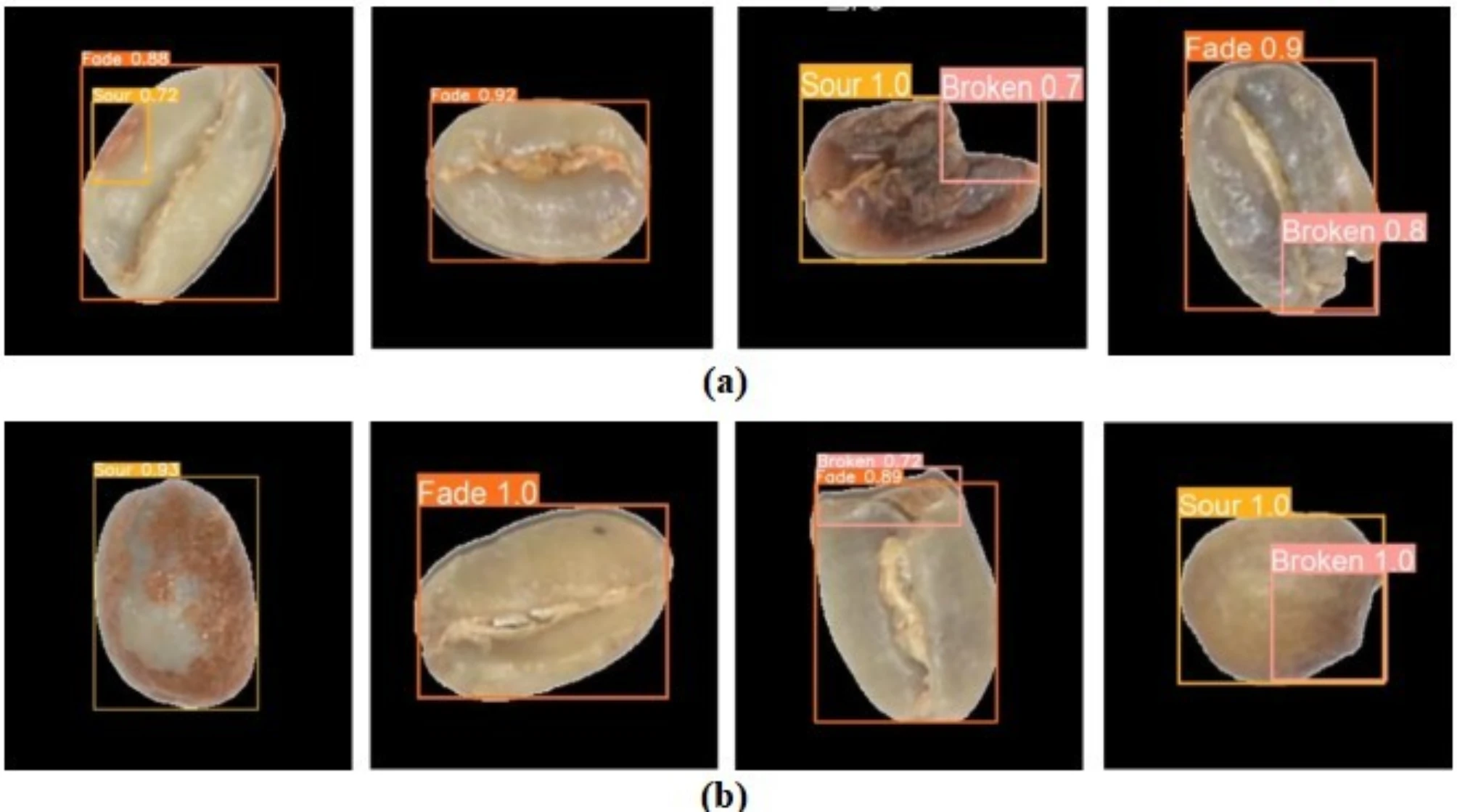
A visual of detection results of a customized computational model for identifying coffee defects.. The use of this image is licensed under a Creative Commons Attribution-NonCommercial-NoDerivatives 4.0 International License. See the original here.
A research team focused on potential real-world AI solutions for agricultural producers says it has created a highly accurate computational model for identifying defects in green coffees.
The group of scientists from Bangladesh and Japan compared multiple versions of customized YOLO (You Only Look Once) models. YOLO is a type of deep learning algorithm for real-time object detection that is already in use in applications such as medical imaging, security cameras and self-driving cars.
The research team said employing YOLO modeling in green coffee production might ultimately benefit green coffee producers and other industry actors who have relied on hand sorting for various quality control tasks.
βDetecting defective beans is vital for improving coffee production processes,β the researchers wrote, adding, βespecially in developing countries like Bangladesh, where it could significantly boost the economy and improve the livelihoods of farmers.β
As of this writing, there was at least one machine already in use in the coffee industry employing AI algorithms for real-time defect detection, the Avercasso CS1 from Taiwan. Its algorithms and hardware remain proprietary.
For the new open-access study, the researchers collected more than 5,000 high-resolution images of green coffee beans, while identifying for defects listed as: black beans, broken beans, faded beans and sour beans.
Those images were used to train six different YOLO models, each of which was then tested for accuracy.
One custom-modified model, called YOLOv8n, stood above the rest of the field in terms of defect identification accuracy. The model also bested other models inΒ minimizing false positives and negatives.
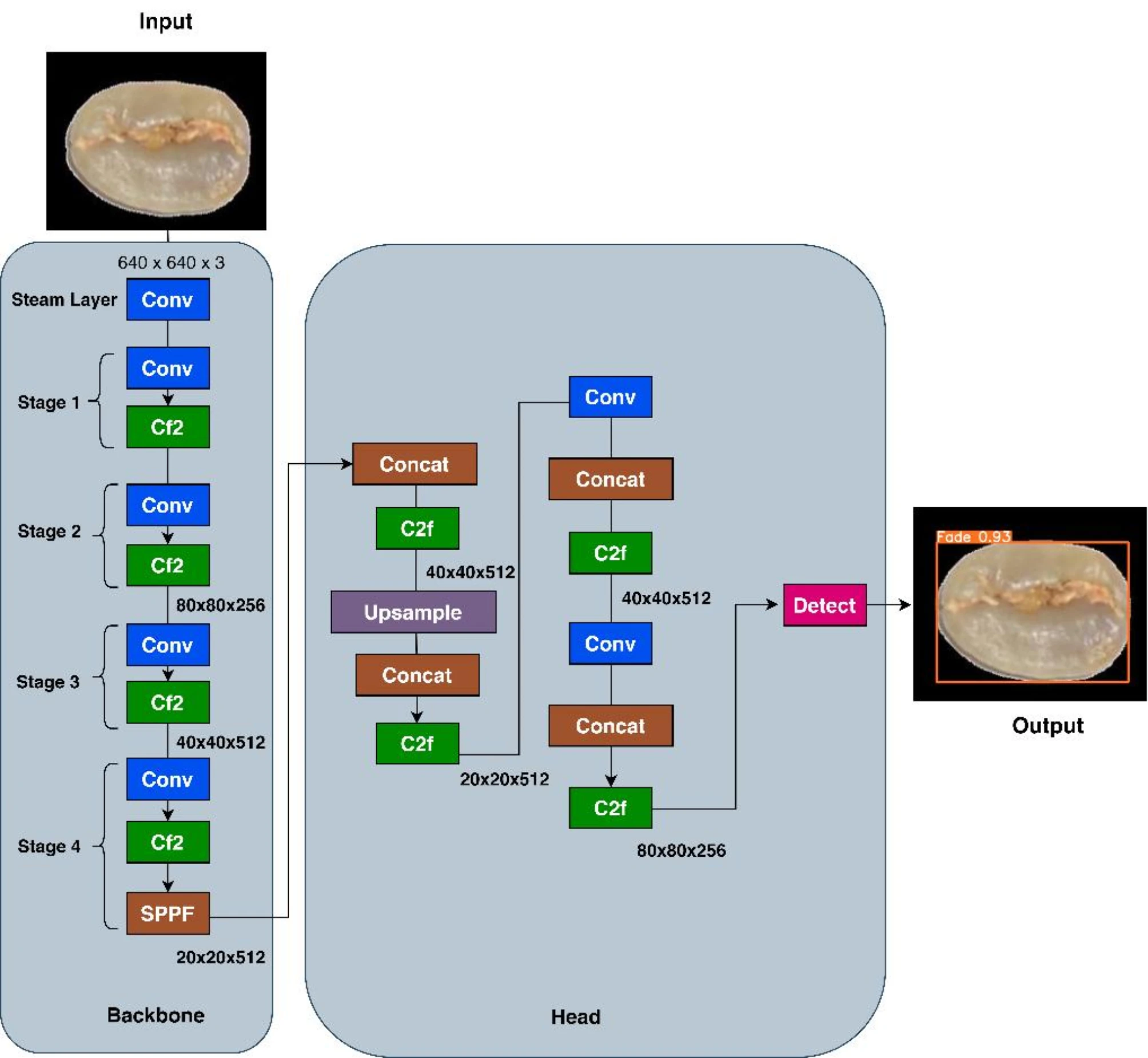
The architecture of the customized YOLOv8n model. This use of this image is licensed under a Creative Commons Attribution-NonCommercial-NoDerivatives 4.0 International License. See the original here.
βThe proposed custom-YOLOv8n model exhibited superior performance across all key metrics when compared to previous versions, suggesting its strong potential for practical applications in coffee bean defect detection,β the study states.
However, the researchers also noted several practical limitations, including significant computational resources required to refine the model and an extensive training process.
Additionally, the study focused on coffees from a single source in Bangladesh, meaning the efficacy of the models may not necessarily translate when applied to different types of coffees from different countries or regions.
βExpanding the dataset to include beans from diverse origins and defect types could improve the modelβs generalizability,β the researchers wrote. βFuture research could also integrate IoT (Internet of Things) devices for real-time monitoring and explore applications beyond coffee, such as detecting defects in other agricultural products.β
The full study, called βComparative analysis of YOLO models for green coffee bean detection and defect classification,β was published in the Nature journalΒ Scientific Reports.Β
Comments? Questions? News to share? Contact DCNβs editors here.Β